MM1076 M.2 M Key Card
Overview
The MM1076 M.2 M key card enables high-performance andpower-efficient AI inference for edge devices and edge servers. The M.2 card’s compact form-factor and popularity makes integration into many different systems a straightforward task. The MM1076 is designed with the M1076 Mythic AMP™ which is arranged in an array of AMP tiles that each feature a Mythic ACE™. The MM1076 is ideal for processing deep neural network (DNN) models in a variety of applications, including video surveillance, industrial machine vision, drone, AR/VR, and edge servers.
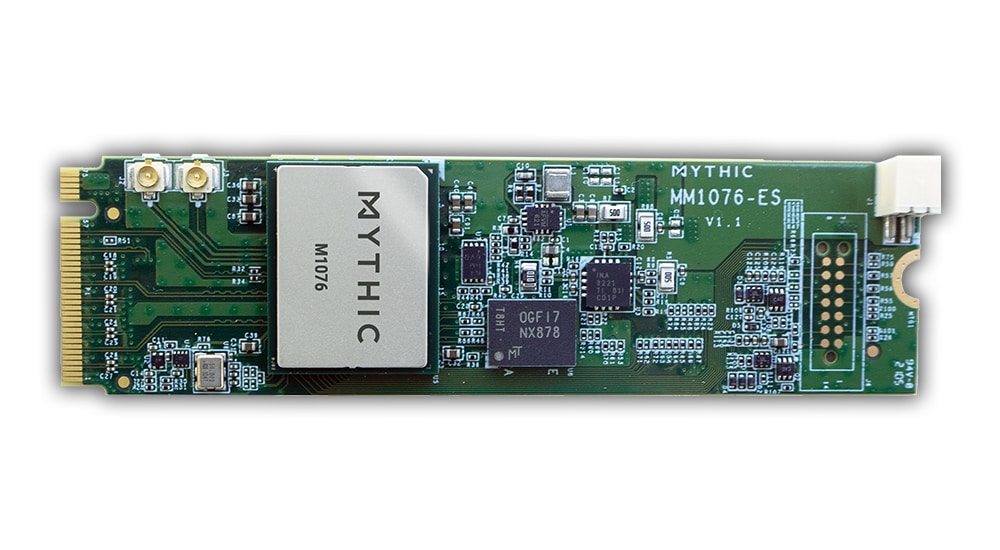
Features
M1076 Mythic AMP™ with support for up to 80M weights on-chip
No external DRAM required
SMBus for EEPROM and PMIC access
Pre-qualified networks including object detectors, classifiers, pose estimators, with more being added
OS Support: Ubuntu, NVIDIA L4T, and Windows (future release)
Model parameters stored and matrix operations executed on-chip by AMP tiles
4-lane PCIe 2.1 for up to 2GB/s bandwidth
Support for standard frameworks, including PyTorch, TensorFlow 2.0, and Caffe
Small 22mm x 80mm form factor
Workflow
DNN models developed in standard frameworks such as Pytorch, Caffe, and TensorFlow are implemented and deployed on the Mythic Analog Matrix Processor (Mythic AMPTM) using Mythic’s AI software workflow. Models are optimized, quantized from FP32 to INT8, and then retrained for the Mythic Analog Compute Engine (Mythic ACETM) prior to being processed through Mythic’s powerful graph compiler. Resultant binaries and model weights are then programmed into the Mythic AMP for inference. Pre-qualified models are also available for developers to quickly evaluate the Mythic AMP solution.
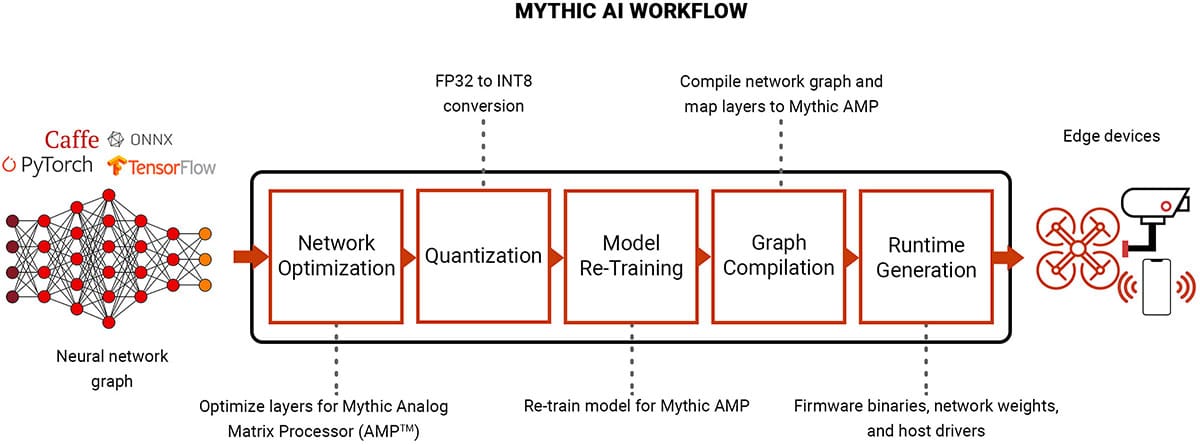
Models
Mythic provides powerful pre-qualified models for the most popular AI use cases. Models have been optimized to take advantage of the high-performance and low-power features of Mythic Analog Matrix Processors (Mythic AMPTM). Developers can focus on model performance and end-application integration instead of the time-consuming model development and training process. Available pre-qualified models in development: